Aryasomayajula, S; Hing, CB; Siebachmeyer, M; Naeini, FB; Ejindu, V; Leitch, P; Gelfer, Y; Zweiri, Y
(2023)
Developing an artificial intelligence diagnostic tool for paediatric distal radius fractures, a proof of concept study.
Annals of the Royal College of Surgeons of England, 105 (8).
pp. 721-728.
ISSN 0035-8843
https://doi.org/10.1308/rcsann.2023.0017
SGUL Authors: Hing, Caroline Blanca
![[img]](https://openaccess.sgul.ac.uk/115221/1.hassmallThumbnailVersion/RCS%20proof%2013.10.22.pdf) 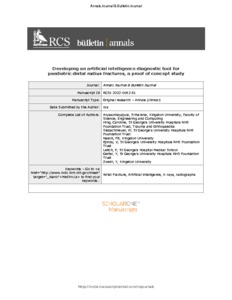 Preview |
|
PDF
Accepted Version
Available under License ["licenses_description_publisher" not defined].
Download (584kB)
| Preview
|
Abstract
Introduction
In the UK, 1 in 50 children sustain a fractured bone yearly, yet studies have shown that 34% of children sustaining an injury do not have a visible fracture on initial radiographs. Wrist fractures are particularly difficult to identify because the growth plate poses diagnostic challenges when interpreting radiographs.
Methods
We developed Convolutional Neural Network (CNN) image recognition software to detect fractures in radiographs from children. A consecutive data set of 5,000 radiographs of the distal radius in children aged less than 19 years from 2014 to 2019 was used to train the CNN. In addition, transfer learning from a VGG16 CNN pretrained on non-radiological images was applied to improve generalisation of the network and the classification of radiographs. Hyperparameter tuning techniques were used to compare the model with the radiology reports that accompanied the original images to determine diagnostic test accuracy.
Results
The training set consisted of 2,881 radiographs with a fracture and 1,571 without; 548 radiographs were outliers. With additional augmentation, the final data set consisted of 15,498 images. The data set was randomly split into three subsets: training (70%), validation (10%) and test (20%). After training for 20 epochs, the diagnostic test accuracy was 85%.
Discussion
A CNN model is feasible in diagnosing paediatric wrist fractures. We demonstrated that this application could be utilised as a tool for improving diagnostic accuracy. Future work would involve developing automated treatment pathways for diagnosis, reducing unnecessary hospital visits and allowing staff redeployment to other areas.
Statistics
Item downloaded times since 03 Mar 2023.
Actions (login required)
 |
Edit Item |