Akstinaite, V; Garrard, P; Sadler-Smith, E
(2022)
Identifying linguistic markers of CEO hubris: a machine learning approach.
BRITISH JOURNAL OF MANAGEMENT, 33 (3).
pp. 1163-1178.
ISSN 1045-3172
https://doi.org/10.1111/1467-8551.12503
SGUL Authors: Garrard, Peter
![[img]](https://openaccess.sgul.ac.uk/113172/1.hassmallThumbnailVersion/BJM_text-combined.pdf) 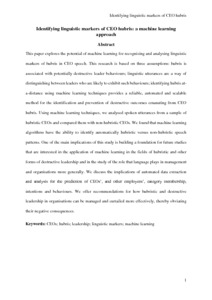 Preview |
|
PDF
Accepted Version
Available under License ["licenses_description_publisher" not defined].
Download (568kB)
| Preview
|
Abstract
This paper explores the potential of machine learning for recognizing and analysing linguistic markers of hubris in CEO speech. This research is based on three assumptions: hubris is associated with potentially destructive leader behaviours; linguistic utterances are a way of distinguishing between leaders who are likely to exhibit such behaviours; identifying hubris at‐a‐distance using machine learning techniques provides a reliable, automated and scalable method for the identification and prevention of destructive outcomes emanating from CEO hubris. Using machine learning techniques, we analysed spoken utterances from a sample of hubristic CEOs and compared them with non‐hubristic CEOs. We found that machine learning algorithms have the ability to identify automatically hubristic versus non‐hubristic speech patterns. One of the main implications of this study is building a foundation for future studies that are interested in the application of machine learning in the fields of hubristic and other forms of destructive leadership, and in the study of the role that language plays in management and organizations more generally. We discuss the implications of automated data extraction and analysis for the prediction of CEOs’, and other employees’, category membership, intentions and behaviours. We offer recommendations for how hubristic and destructive leadership in organizations can be managed and curtailed more effectively, thereby obviating their negative consequences.
Statistics
Item downloaded times since 19 Apr 2021.
Actions (login required)
 |
Edit Item |