Ratzinger, F;
Bruckschwaiger, H;
Wischenbart, M;
Parschalk, B;
Fernandez-Reyes, D;
Lagler, H;
Indra, A;
Graninger, W;
Winkler, S;
Krishna, S;
et al.
Ratzinger, F; Bruckschwaiger, H; Wischenbart, M; Parschalk, B; Fernandez-Reyes, D; Lagler, H; Indra, A; Graninger, W; Winkler, S; Krishna, S; Ramharter, M
(2012)
Rapid Diagnostic Algorithms as a Screening Tool for Tuberculosis: An Assessor Blinded Cross-Sectional Study.
PLOS ONE, 7 (11).
e49658 (1)- e49658 (6).
ISSN 1932-6203
https://doi.org/10.1371/journal.pone.0049658
SGUL Authors: Krishna, Sanjeev
![[img]](https://openaccess.sgul.ac.uk/107060/1.hassmallThumbnailVersion/pone.0049658.pdf) 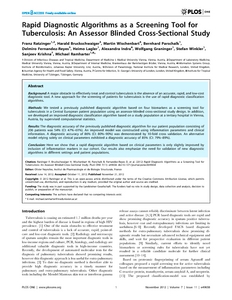 Preview |
|
["document_typename_application/pdf; charset=binary" not defined]
Published Version
Available under License St George's repository terms & conditions.
Download (97kB)
| Preview
|
Abstract
Background: A major obstacle to effectively treat and control tuberculosis is the absence of an accurate, rapid, and low-cost diagnostic tool. A new approach for the screening of patients for tuberculosis is the use of rapid diagnostic classification algorithms.
Methods: We tested a previously published diagnostic algorithm based on four biomarkers as a screening tool for
tuberculosis in a Central European patient population using an assessor-blinded cross-sectional study design. In addition, we developed an improved diagnostic classification algorithm based on a study population at a tertiary hospital in Vienna, Austria, by supervised computational statistics.
Results: The diagnostic accuracy of the previously published diagnostic algorithm for our patient population consisting of 206 patients was 54% (CI: 47%–61%). An improved model was constructed using inflammation parameters and clinical information. A diagnostic accuracy of 86% (CI: 80%–90%) was demonstrated by 10-fold cross validation. An alternative model relying solely on clinical parameters exhibited a diagnostic accuracy of 85% (CI: 79%–89%).
Conclusion: Here we show that a rapid diagnostic algorithm based on clinical parameters is only slightly improved by
inclusion of inflammation markers in our cohort. Our results also emphasize the need for validation of new diagnostic algorithms in different settings and patient populations.
Item Type: |
Article
|
Additional Information: |
Copyright: 2012 Ratzinger et al. This is an open-access article distributed under the terms of the Creative Commons Attribution License, which permits unrestricted use, distribution, and reproduction in any medium, provided the original author and source are credited |
Keywords: |
Adult, Aged, Algorithms, Artificial Intelligence, Biological Markers, Cohort Studies, Cross-Sectional Studies, Double-Blind Method, Female, Humans, Inflammation, Male, Mass Screening, Middle Aged, Reproducibility of Results, Tuberculosis, Science & Technology, Multidisciplinary Sciences |
SGUL Research Institute / Research Centre: |
Academic Structure > Infection and Immunity Research Institute (INII) |
Journal or Publication Title: |
PLOS ONE |
ISSN: |
1932-6203 |
Related URLs: |
|
Dates: |
Date | Event |
---|
21 November 2012 | Published |
|
Web of Science ID: |
WOS:000311821000077 |
URI: |
https://openaccess.sgul.ac.uk/id/eprint/107060 |
Publisher's version: |
https://doi.org/10.1371/journal.pone.0049658 |
Statistics
Item downloaded times since 17 Jul 2014.
Actions (login required)
 |
Edit Item |